Multivariate machine learning algorithms for real-time power plant component life prediction (ML-Real-Time)
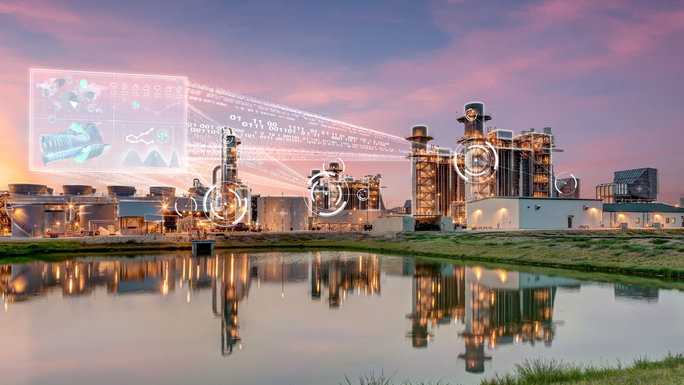
In the ML-Real-Time project (Multivariate machine learning algorithms for real-time power plant component life prediction), Hochschule Niederrhein aims to increase the efficiency of new and existing power plants and reduce the consumption of components. To this end, the project team combines machine learning (ML) methods and stochastic analysis as well as optimal control into a new research approach. The project is led by Prof. Dr.-Ing. Dirk Roos.
Challenge
In view of climate change, technologies for an economical, efficient, and secure energy supply are of crucial importance. In order to reconcile economic efficiency and environmental compatibility, power plant technology must be continuously developed. This involves optimizing existing plants as well as integrating renewable energy sources and small-scale plants such as combined heat and power plants into the grid infrastructure. However, the increasing share of electricity from renewables is raising the demands on the operation of conventional power plants, and conventional design methods are proving increasingly uneconomical. Simulating the complex changing processes in the power grid usually requires a high computational effort, which, however, can be significantly reduced if appropriate efficient ML metamodels for optimal control are used. Here, each optimization variable represents a locally and spatially distributed function. Thus, integral objectives such as lifetime consumption in the current start-stop cycle can be pursued by predicting future condition data from the ML model in real time.
Goals and procedure
The aim of the ML-Real-Time project is to increase the efficiency of new and existing power plants and to reduce the lifetime consumption of plant components. The machine learning algorithms required for the optimal design and real-time service life prediction of components will then be implemented in existing software for optimization and stochastic analysis at the Institute for Modelling and High-Performance Computing at Hochschule Niederrhein. In another work package of the project, the so-called Gaussian covariance networks will be extended for application in optimal control. Short training times of the ML algorithms as well as real-time capability are to be ensured by installing the software on a GPU (Graphics Processing Unit) cluster for HPC (High Performance Computing). Thus, the system will be able to react to changes in the input parameters with immediate effect.
Innovations and perspectives
The machine learning approaches developed in the project enable the ad-hoc optimization of power plants, so that they can be applied to a large number of existing plants. The cooperation partner Siemens Energy AG will utilize the results early on to increase efficiency, make power plant operation more flexible, and reduce pollutant emissions. This will involve the development of more efficient, more cost-effective and, above all, more durable power plant components, as well as the potential optimization of other technical systems. However, the developments are not only interesting for power plant development, but can also be used in Industry 4.0, for example for the digitalization and optimization of product development processes. The project partners therefore consider the exploitation potential of the expected results to be very high.