High-precision non-destructive testing in industrial production using new AI methods (NextGenNDT)
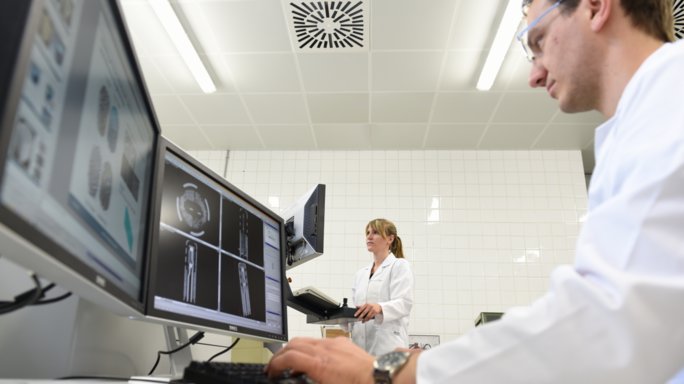
In the NextGenNDT project (High-precision non-destructive testing in industrial production using new AI methods), Aalen University is developing a method for non-destructive testing (NDT) based on artificial intelligence (AI) that can be used in industrial-scale production and assists human inspectors. The project is led by Prof. Dr. Ricardo Büttner.
Challenge
Non-destructive material testing (NDT) is a tool used for quality control in industrial production. Its main advantage is that the component to be tested is not damaged and can still be used or sold afterwards. However, conventional NDT also has some problems: As defect detection has hardly been automated so far, it is mostly carried out on random samples by human inspectors. These manual examinations are not always precise and the results vary depending on the inspector, so that defects can remain undetected. Moreover, the 3D recording techniques used in quality control generate a large amount of data. This can only be handled with enormous human effort. Therefore, a ubiquitous use of NDT inspection in mass production is hardly feasible from an economic and technical point of view but would actually be necessary to ensure high product quality.
Goals and procedure
The NextGenNDT project aims to develop highly accurate, fast methods of automatic defect detection in non-destructive 3D material testing that are applicable on an industrial scale. To this end, the project team develops powerful deep learning methods in several steps. First, training data is collected and checked for defects (labelling). Different AI architectures are then trained and tested based on this data and the most promising candidates are optimized. The convolutional neural networks (ConvNets) used in the project are particularly suitable for transfer learning, a process in which algorithms are trained on a large training data set. After this initial training with large amounts of data has been completed, the network can be fine-tuned to smaller data sets. This allows the ConvNets to be readily transferred to other testing tasks. Transferability is supported by the development of a standardized application programming interface (API).
Innovations and perspectives
The NextGenNDT project uses state-of-the-art machine learning algorithms for in-process defect detection; this has not been realized in non-destructive 3D component testing so far. The methods can be easily transferred to other applications via standardized program interfaces, enabling a widespread application of non-destructive component testing in mass production and also mapping undetected defects in production processes. The project results benefit industrial users who can integrate them into their own testing processes as well as scientists who can further develop their deep learning methods. In addition, the project gives students an insight into industry-relevant research projects and potential solutions in the field of AI research.